
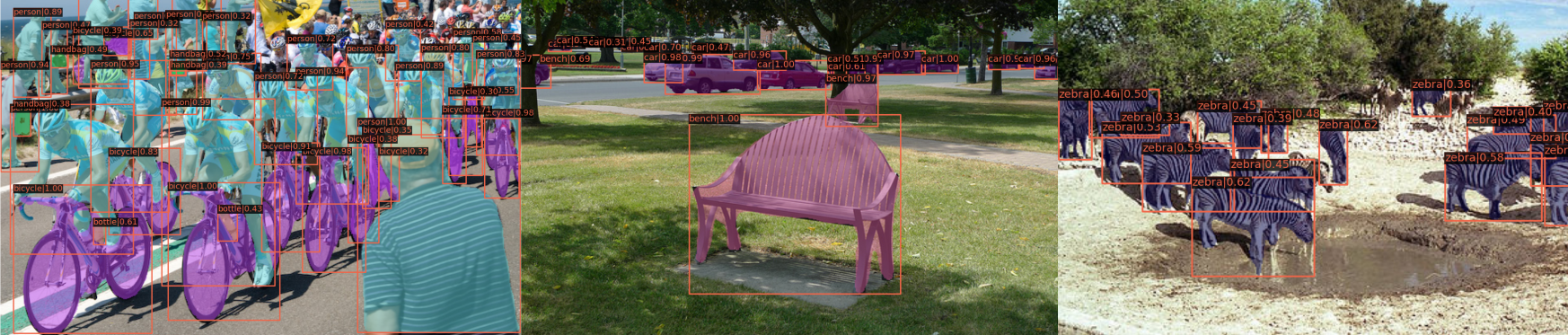
Introduction
English | 简体中文
MMDetection is an open source object detection toolbox based on PyTorch. It is a part of the OpenMMLab project.
The master branch works with PyTorch 1.3+.
Major features
-
Modular Design
We decompose the detection framework into different components and one can easily construct a customized object detection framework by combining different modules.
-
Support of multiple frameworks out of box
The toolbox directly supports popular and contemporary detection frameworks, e.g. Faster RCNN, Mask RCNN, RetinaNet, etc.
-
High efficiency
All basic bbox and mask operations run on GPUs. The training speed is faster than or comparable to other codebases, including Detectron2, maskrcnn-benchmark and SimpleDet.
-
State of the art
The toolbox stems from the codebase developed by the MMDet team, who won COCO Detection Challenge in 2018, and we keep pushing it forward.
Apart from MMDetection, we also released a library mmcv for computer vision research, which is heavily depended on by this toolbox.
License
This project is released under the Apache 2.0 license.
Changelog
2.18.0 was released in 27/10/2021:
- Support QueryInst.
- Refactor dense_head to decouple onnx export from get_bboxes and speed up inference time.
- Support infinite sampler to accelerate training when using iter based runner.
Please refer to changelog.md for details and release history.
For compatibility changes between different versions of MMDetection, please refer to compatibility.md.
Benchmark and model zoo
Results and models are available in the model zoo.
Supported backbones:
- ResNet (CVPR'2016)
- ResNeXt (CVPR'2017)
- VGG (ICLR'2015)
- MobileNetV2 (CVPR'2018)
- HRNet (CVPR'2019)
- RegNet (CVPR'2020)
- Res2Net (TPAMI'2020)
- ResNeSt (ArXiv'2020)
- Swin (CVPR'2021)
- PVT (ICCV'2021)
- PVTv2 (ArXiv'2021)
Supported methods:
- RPN (NeurIPS'2015)
- Fast R-CNN (ICCV'2015)
- Faster R-CNN (NeurIPS'2015)
- Mask R-CNN (ICCV'2017)
- Cascade R-CNN (CVPR'2018)
- Cascade Mask R-CNN (CVPR'2018)
- SSD (ECCV'2016)
- RetinaNet (ICCV'2017)
- GHM (AAAI'2019)
- Mask Scoring R-CNN (CVPR'2019)
- Double-Head R-CNN (CVPR'2020)
- Hybrid Task Cascade (CVPR'2019)
- Libra R-CNN (CVPR'2019)
- Guided Anchoring (CVPR'2019)
- FCOS (ICCV'2019)
- RepPoints (ICCV'2019)
- Foveabox (TIP'2020)
- FreeAnchor (NeurIPS'2019)
- NAS-FPN (CVPR'2019)
- ATSS (CVPR'2020)
- FSAF (CVPR'2019)
- PAFPN (CVPR'2018)
- Dynamic R-CNN (ECCV'2020)
- PointRend (CVPR'2020)
- CARAFE (ICCV'2019)
- DCNv2 (CVPR'2019)
- Group Normalization (ECCV'2018)
- Weight Standardization (ArXiv'2019)
- OHEM (CVPR'2016)
- Soft-NMS (ICCV'2017)
- Generalized Attention (ICCV'2019)
- GCNet (ICCVW'2019)
- Mixed Precision (FP16) Training (ArXiv'2017)
- InstaBoost (ICCV'2019)
- GRoIE (ICPR'2020)
- DetectoRS (ArXiv'2020)
- Generalized Focal Loss (NeurIPS'2020)
- CornerNet (ECCV'2018)
- Side-Aware Boundary Localization (ECCV'2020)
- YOLOv3 (ArXiv'2018)
- PAA (ECCV'2020)
- YOLACT (ICCV'2019)
- CentripetalNet (CVPR'2020)
- VFNet (ArXiv'2020)
- DETR (ECCV'2020)
- Deformable DETR (ICLR'2021)
- CascadeRPN (NeurIPS'2019)
- SCNet (AAAI'2021)
- AutoAssign (ArXiv'2020)
- YOLOF (CVPR'2021)
- Seasaw Loss (CVPR'2021)
- CenterNet (CVPR'2019)
- YOLOX (ArXiv'2021)
- SOLO (ECCV'2020)
- QueryInst (ICCV'2021)
Some other methods are also supported in projects using MMDetection.
Installation
Please refer to get_started.md for installation.
Getting Started
Please see get_started.md for the basic usage of MMDetection. We provide colab tutorial, and full guidance for quick run with existing dataset and with new dataset for beginners. There are also tutorials for finetuning models, adding new dataset, designing data pipeline, customizing models, customizing runtime settings and useful tools.
Please refer to FAQ for frequently asked questions.
Contributing
We appreciate all contributions to improve MMDetection. Ongoing projects can be found in out GitHub Projects. Welcome community users to participate in these projects. Please refer to CONTRIBUTING.md for the contributing guideline.
Acknowledgement
MMDetection is an open source project that is contributed by researchers and engineers from various colleges and companies. We appreciate all the contributors who implement their methods or add new features, as well as users who give valuable feedbacks. We wish that the toolbox and benchmark could serve the growing research community by providing a flexible toolkit to reimplement existing methods and develop their own new detectors.
Citation
If you use this toolbox or benchmark in your research, please cite this project.
@article{mmdetection,
title = {{MMDetection}: Open MMLab Detection Toolbox and Benchmark},
author = {Chen, Kai and Wang, Jiaqi and Pang, Jiangmiao and Cao, Yuhang and
Xiong, Yu and Li, Xiaoxiao and Sun, Shuyang and Feng, Wansen and
Liu, Ziwei and Xu, Jiarui and Zhang, Zheng and Cheng, Dazhi and
Zhu, Chenchen and Cheng, Tianheng and Zhao, Qijie and Li, Buyu and
Lu, Xin and Zhu, Rui and Wu, Yue and Dai, Jifeng and Wang, Jingdong
and Shi, Jianping and Ouyang, Wanli and Loy, Chen Change and Lin, Dahua},
journal= {arXiv preprint arXiv:1906.07155},
year={2019}
}
Projects in OpenMMLab
- MMCV: OpenMMLab foundational library for computer vision.
- MIM: MIM Installs OpenMMLab Packages.
- MMClassification: OpenMMLab image classification toolbox and benchmark.
- MMDetection: OpenMMLab detection toolbox and benchmark.
- MMDetection3D: OpenMMLab's next-generation platform for general 3D object detection.
- MMSegmentation: OpenMMLab semantic segmentation toolbox and benchmark.
- MMAction2: OpenMMLab's next-generation action understanding toolbox and benchmark.
- MMTracking: OpenMMLab video perception toolbox and benchmark.
- MMPose: OpenMMLab pose estimation toolbox and benchmark.
- MMEditing: OpenMMLab image and video editing toolbox.
- MMOCR: A Comprehensive Toolbox for Text Detection, Recognition and Understanding.
- MMGeneration: OpenMMLab image and video generative models toolbox.