代码版本:0714 commit #4000
$ git clone https://github.com/ultralytics/yolov5
$ cd yolov5
$ git checkout 720aaa65c8873c0d87df09e3c1c14f3581d4ea61
这个代码只是注释版本哈,不是很建议直接运行。
考虑到可能会有人直接运行,可能之后有时间会出一个新的6.0版本的注释emmmm.
- yolov5-6.0
最近发现了一个更详细的yolov5
注释版yolov5-5.x-annotations.
下面的文件均有注释,有些没有用到的函数,以及网络结构模块等没有注释
yolov5_annotations
├── data
├── models
│ ├── common.py
│ ├── experimental.py
│ ├── yolo.py
├── utils
│ ├── augmentations.py
│ ├── autoanchor.py
│ ├── datasets.py
│ ├── general.py
│ ├── loss.py
│ ├── metrics.py
│ ├── plots.py
│ ├── torch_utils.py
│ ├── google_utils.py
├── export.py
├── hubconf.py
├── train.py
├── val.py
├── detect.py
原README分割线
YOLOv5
Documentation
See the YOLOv5 Docs for full documentation on training, testing and deployment.
Quick Start Examples
Install
Python >= 3.6.0 required with all requirements.txt dependencies installed:
$ git clone https://github.com/ultralytics/yolov5
$ cd yolov5
$ pip install -r requirements.txt
Inference
Inference with YOLOv5 and PyTorch Hub. Models automatically download from the latest YOLOv5 release.
import torch
# Model
model = torch.hub.load('ultralytics/yolov5', 'yolov5s') # or yolov5m, yolov5x, custom
# Images
img = 'https://ultralytics.com/images/zidane.jpg' # or file, PIL, OpenCV, numpy, multiple
# Inference
results = model(img)
# Results
results.print() # or .show(), .save(), .crop(), .pandas(), etc.
Inference with detect.py
detect.py
runs inference on a variety of sources, downloading models automatically from the latest YOLOv5 release and saving results to runs/detect
.
$ python detect.py --source 0 # webcam
file.jpg # image
file.mp4 # video
path/ # directory
path/*.jpg # glob
'https://youtu.be/NUsoVlDFqZg' # YouTube video
'rtsp://example.com/media.mp4' # RTSP, RTMP, HTTP stream
Training
Run commands below to reproduce results on COCO dataset (dataset auto-downloads on first use). Training times for YOLOv5s/m/l/x are 2/4/6/8 days on a single V100 (multi-GPU times faster). Use the largest --batch-size
your GPU allows (batch sizes shown for 16 GB devices).
$ python train.py --data coco.yaml --cfg yolov5s.yaml --weights '' --batch-size 64
yolov5m 40
yolov5l 24
yolov5x 16
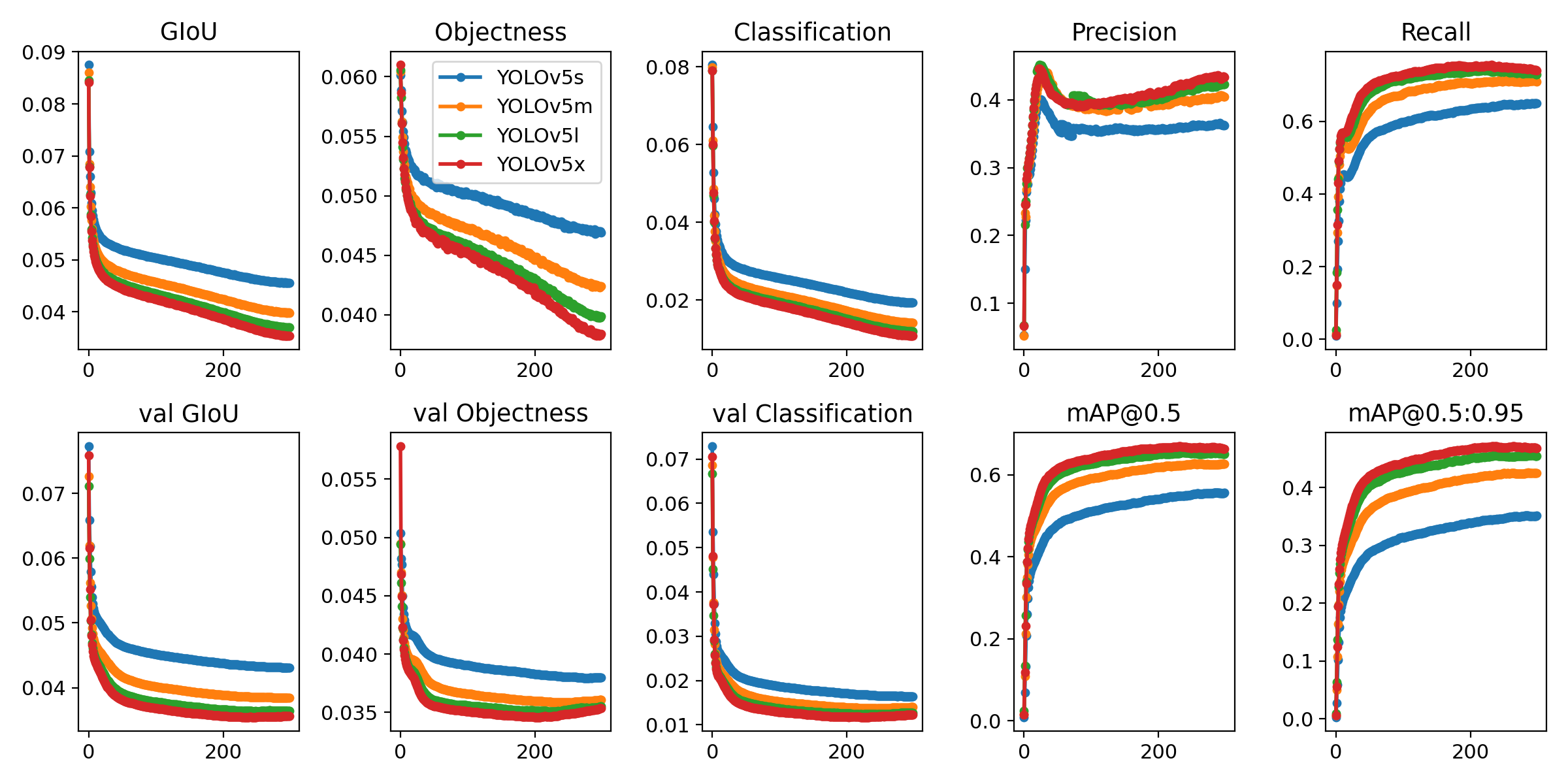
Tutorials
- Train Custom Data
🚀 RECOMMENDED - Tips for Best Training Results
☘️ RECOMMENDED - Weights & Biases Logging
🌟 NEW - Supervisely Ecosystem
🌟 NEW - Multi-GPU Training
- PyTorch Hub
⭐ NEW - TorchScript, ONNX, CoreML Export
🚀 - Test-Time Augmentation (TTA)
- Model Ensembling
- Model Pruning/Sparsity
- Hyperparameter Evolution
- Transfer Learning with Frozen Layers
⭐ NEW - TensorRT Deployment
Environments and Integrations
Get started in seconds with our verified environments and integrations, including Weights & Biases for automatic YOLOv5 experiment logging. Click each icon below for details.
Compete and Win
We are super excited about our first-ever Ultralytics YOLOv5 🚀 EXPORT Competition with $10,000 in cash prizes!
Why YOLOv5
Figure Notes (click to expand)
- GPU Speed measures end-to-end time per image averaged over 5000 COCO val2017 images using a V100 GPU with batch size 32, and includes image preprocessing, PyTorch FP16 inference, postprocessing and NMS.
- EfficientDet data from google/automl at batch size 8.
- Reproduce by
python val.py --task study --data coco.yaml --iou 0.7 --weights yolov5s6.pt yolov5m6.pt yolov5l6.pt yolov5x6.pt
Pretrained Checkpoints
Model | size (pixels) |
mAPval 0.5:0.95 |
mAPtest 0.5:0.95 |
mAPval 0.5 |
Speed V100 (ms) |
params (M) |
FLOPs 640 (B) |
|
---|---|---|---|---|---|---|---|---|
YOLOv5s | 640 | 36.7 | 36.7 | 55.4 | 2.0 | 7.3 | 17.0 | |
YOLOv5m | 640 | 44.5 | 44.5 | 63.1 | 2.7 | 21.4 | 51.3 | |
YOLOv5l | 640 | 48.2 | 48.2 | 66.9 | 3.8 | 47.0 | 115.4 | |
YOLOv5x | 640 | 50.4 | 50.4 | 68.8 | 6.1 | 87.7 | 218.8 | |
YOLOv5s6 | 1280 | 43.3 | 43.3 | 61.9 | 4.3 | 12.7 | 17.4 | |
YOLOv5m6 | 1280 | 50.5 | 50.5 | 68.7 | 8.4 | 35.9 | 52.4 | |
YOLOv5l6 | 1280 | 53.4 | 53.4 | 71.1 | 12.3 | 77.2 | 117.7 | |
YOLOv5x6 | 1280 | 54.4 | 54.4 | 72.0 | 22.4 | 141.8 | 222.9 | |
YOLOv5x6 TTA | 1280 | 55.0 | 55.0 | 72.0 | 70.8 | - | - |
Table Notes (click to expand)
- APtest denotes COCO test-dev2017 server results, all other AP results denote val2017 accuracy.
- AP values are for single-model single-scale unless otherwise noted. Reproduce mAP by
python val.py --data coco.yaml --img 640 --conf 0.001 --iou 0.65
- SpeedGPU averaged over 5000 COCO val2017 images using a GCP n1-standard-16 V100 instance, and includes FP16 inference, postprocessing and NMS. Reproduce speed by
python val.py --data coco.yaml --img 640 --conf 0.25 --iou 0.45
- All checkpoints are trained to 300 epochs with default settings and hyperparameters (no autoaugmentation).
- Test Time Augmentation (TTA) includes reflection and scale augmentation. Reproduce TTA by
python val.py --data coco.yaml --img 1536 --iou 0.7 --augment
Contribute
We love your input! We want to make contributing to YOLOv5 as easy and transparent as possible. Please see our Contributing Guide to get started.
Contact
For issues running YOLOv5 please visit GitHub Issues. For business or professional support requests please visit https://ultralytics.com/contact.